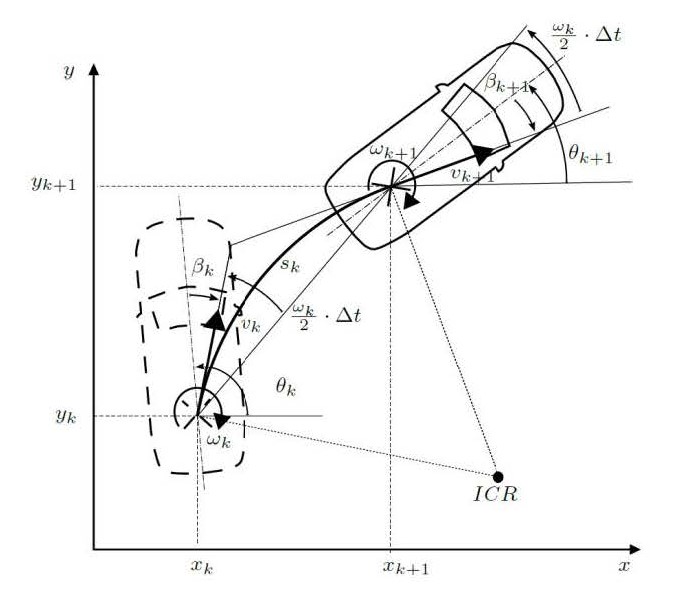
Odometry 2.0: A Slip-Adaptive EIF-Based Four-Wheel-Odometry Model for Parking
-
Autor:
Wohlgemuth, T.
-
Quelle:
IEEE Transactions on Intelligent Vehicles,
New York, USA
DOI: 10.1109/TIV.2018.2886675 - Datum: März 2019
-
This paper investigates the possibility of estimating the actual pose of a passenger car while parking by adaptive multi-sensor data fusion. The newly developed Odometry 2.0 estimator includes multiple dead-reckoning models implemented by the adaptive measurement model of an Extended Information filter (EIF). The measurements of orientation and velocity of each of the four wheels, corrected kinematic side-slip angles and the yaw rate serve as input for the estimator. The chosen architecture allows the measurements to be combined individually. This makes it possible to deal with systematic errors in modelling, depending on the quality of the measurements, by changing the composition of the measurement model. In addition, a Slip Detection Algorithm (SDA) is implemented, which sets the information measurement covariance of the slipping wheel measurement to 0, so that the inaccurate measurement cannot influence the estimated pose. Separate modeling of translation and rotation allows to estimate the vehicle's position from the information of a single wheel, making it a true four-wheel-odometry model. Experimental results confirm the excellent performance of the Odometry 2.0 estimator in contrast to the well-known state-of-the-art dead-rocking models for parking, analyzed by position data obtained from a Dual-DGNSS-IMU reference system. The SDA was tested under real conditions on partially snow-covered areas.